Table of Contents
The credibility of a brand is built through the reliable delivery of an experience as well as presenting a consistent image that matches brand values. Both hinge on the quality of product information and images that may be seen in any number of places — on e-commerce sites, the brand’s website, in promotional flyers, packaging, on in-store signs or displays, advertisements, social sites, and more. Establishing a source of truth for product content and protecting brand integrity is crucial to reinforce image and trust among consumers and retailer partners.
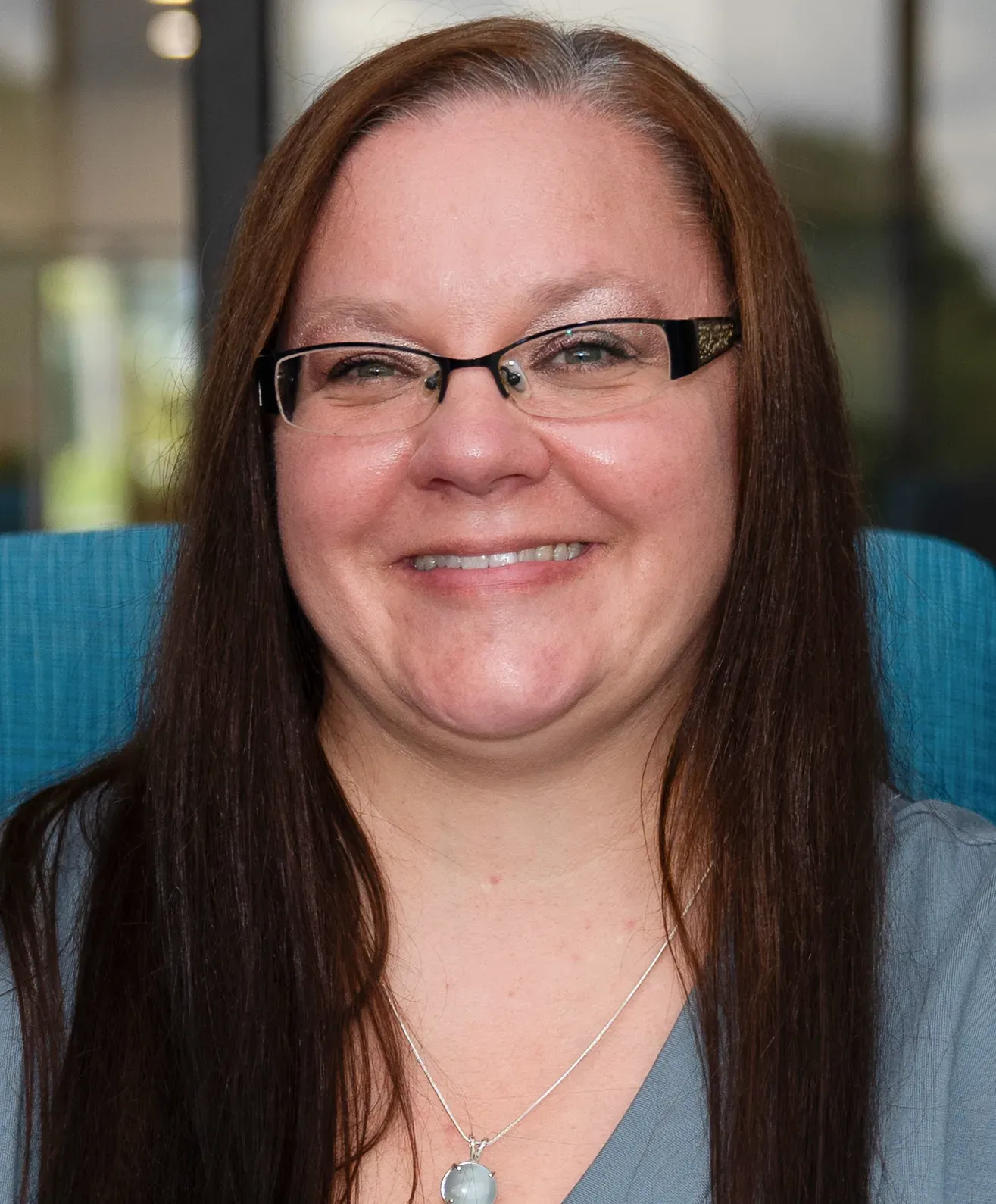
If you’re in the business of product information management or data asset management, you’re very aware of the importance of a source of truth for current, accurate product content. And, in an effort to streamline the capture of product content, you likely have looked into or started to use artificial intelligence (AI). AI can make an otherwise highly tactical process more efficient and streamlined. But don’t expect AI to be a total solution. Like most uses of AI, human intervention is still necessary, but certainly there are productivity gains to be had.
To start, understand the background of the AI tool being used to enter product content information. Has it been used for similar products? Think not only on a broad level, such as food versus health, beauty, and wellness (HBW) items, but also consider the category, subcategory, and segment levels it has been introduced to. If the platform hasn’t been used for like products, expect a longer period of trial and error. For instance, if you are starting to work with a tool that previously has primarily been used to capture product content for food, there may be corrections needed when scents and flavors in HBW products resemble ingredients in food. We encountered this situation with witch hazel which was overwritten by AI to hazelnut, an ingredient the platform was already familiar with.
Here are some best practices that can make the utilization of AI in product content capture go more smoothly:
- Provide demonstrations for both the AI software team and the PIM/DAM product analysts. While product demos no doubt occur during the sales cycle, having the PIM/DAM product analysts precisely walk through current processes, and giving these experts a view of the platform will help in writing effective business rules and save time during implementation. Both teams can also align to ensure realistic expectations, which is necessary for the next step, building a plan. These initial discussions can also bring to light terminology differences which are helpful to understand up front. For example, when it comes to data, the definition of “field” can vary depending on perspective.
- Develop a project plan. Even with the demonstrations of processes and platforms, expect that unplanned issues will arise, but putting thought into the best methods and procedures that will make the implementation of the AI tool effective will increase the odds of achieving success. Try to foresee as many issues as possible and think of unintended consequences to prepare for a smoother transition.
- Schedule a regular touch base meeting to discuss issues and clarify needs. These video or in-person meetings can accelerate the understanding of the challenges and determine resolutions more quickly and easily than numerous emails. Not to discount email — that comes in handy when needing to get answers in between meetings and provides a communication trail that can be a great future reference.
- Plan for failure. That’s not as harsh as it sounds, just expect a fair amount of trial and error and remain flexible to accommodate it. As you get a better understanding of how the AI software learns from the data you provide, you may find that providing a subset of data is more effective than a broader range, or you may want to scale back data attributes provided or add some for better results. Also, be sure to provide data you have corrected back to your AI partner to help refine the capabilities of the platform by giving it examples of what is desired.
Machine learning is ongoing and AI software will continually need to be retrained, because there will always be new ingredients, scents, forms, and more for it to learn. Regulations, laws, and guidelines also get refined, therefore AI will have to relearn inputs based on those changes. The smarter it gets the more useful it becomes.
Lisa Lopez is a 20-year retail industry veteran who manages Hammacher Resource Inc.’s (HRG’s) data assets team and is responsible for the processes and procedures in HRG’s capture and quality control of product information and images.